ON THIS EPISODE OF HIGH IMPACT GROWTH
What’s New in Al: Equity-enhancing use cases and Open Chat Studio
LISTEN
Transcript
This transcript was generated by AI and may contain typos and inaccuracies.
Amie Vaccaro: Welcome to the podcast guys. So I’m here with Jonathan Jackson, my cohost. Hey, Jon.
Jonathan Jackson: Hey, Amie,
Amie Vaccaro: And we have today, Brian DiRenzi with us, who you’ve heard from on a couple other episodes namely on AI. And Brian leads our research and data team and has been really leading the charge on a lot of our AI efforts at Dimagi.
Brian DeRenzi: Thanks, Amie. Happy to be here.
Jonathan Jackson: welcome back.
Brian DeRenzi: Thanks, Jon.
Amie Vaccaro: Welcome back to the podcast. It has been almost six months since we published our last conversation about AI. And in that time, I was out on maternity leave for six months and really not thinking about AI. It’s funny to go from just so consumed by something to like, just not even thinking about it.
But now that I’m back at Dimagi, I’m very interested to just hear where you guys heads are at, what you’ve learned, how things have been going, what are we up to and really just catch up on all that’s been going on. It does seem like it’s been a very busy time in the AI world here at Dimagi, and certainly outside of Dimagi as well.
So yeah, maybe just at the highest level, can you catch me up a bit on what you guys have been up to?
Jonathan Jackson: Yeah. Brian, you want to take it away?
Brian DeRenzi: Sure. Maybe I’ll tell one quick story to illustrate things which is I was talking to a partner today about some work that we did with them back in December. And I mentioned that there had been four major releases of the model since we did the original work. And some of the limitations that we put in around the original work were no longer true.
because of some of the new models that came out. It was an interesting thing where I was presenting the work that we had previously done and talking about where it goes and talking about the future. And it’s still so hard to predict because things are moving quickly.
There’s, I think there’s been some talk that things in AI are slowing down, but I think they’re just shifting and the types of improvements that we’re seeing are. Different than the improvements that we were seeing previously. But it, it was an interesting moment where I, started to count the number of model releases between doing the work and the presentation today.
And I was surprised to see how much things have shifted just in, in the last four or five months.
Jonathan Jackson: Yeah. And I think from our our own Dimagi experience, obviously the world of AI is moving extremely fast. The, massive billion dollar foundational model companies are. moving as quickly as they can. Google, Apple, Microsoft, Facebook, Salesforce, they’re all embedding or creating foundational models as quickly as they can.
So it’s very clear the generative AI at a foundational level is going to be in the core tools we’re all used to using. As quickly as possible. If you’re on WhatsApp, there’s already a WhatsApp meta AI you can talk to now. So it’s starting to come out like in the, the mass billion plus user consumer products in a very real way that was envisioned six months ago, but not quite there yet.
And now, Microsoft’s talking about putting it is in their iOS. Apple’s about to embed something in their iOS. So there’s these core. Capabilities that’ll be available to everyone and for Dimagi, we are still racing, as fast as we can towards equitable AI saying, okay, that’s great for the people who are already on the tech curve and already, getting buying MacBook pros and exposed to these tools and technologies that’ll take care of itself.
The market’s driving that, very fast and, seeing prospectuses on up and coming foundational model companies who are, saying, if we get a. 5 percent market share, that’s a trillion dollar company, so they’re like, they’re not claiming they’re going to be the foundational model.
They’re like, even a slice of that kind of core foundational market is going to be a trillion dollar company. So the market is clearly, investing heavily in those companies and that’ll continue to happen. The use cases above those are also racing ahead. We’ve seen tons of progress in medical AI and legal AI and business workflow AI.
We’re going to continue to see rapid progression. I anticipate they are going to get notably better than humans pretty quickly in some types of tasks. One of the things that we’re doing now that Brian’s leading with our team is looking at multi agent. use cases where we’re saying, an AI is going to get, you can make an AI really good at answering a very specific set of questions, but maybe it’s not good at answering every question you could throw at it.
So if you have a family planning question or you have a TB care question, maybe the same bot can answer both of those well, but maybe you want two different bots or agents that can answer those independently once you detect the question that’s being asked. So we’re really excited to explore those multi agent use cases.
And then we’ve really pushed hard into our research and our work on low resource languages. So the models are getting quite good at being, useful in languages that you wouldn’t think. Brian can go into some detail on this. And that’s really exciting because that’s a huge barrier to testing.
There’s the user experience and the language experience of interacting with the model. And then there’s What it’s trying to convey and the what it’s trying to convey in English is already crazy impressive in terms of empathy and response and, almost being better in some ways than a human would be at helping you think through problems or talking to you about certain issues.
But if it only works in English and major languages, then it’s going to not reach probably some of the most important equity use cases. So we’re really excited, but we’re already seeing, and I think there’s gonna be a lot more progress on that ahead as well. Lots of huge areas, and we’ve been very fortunate to receive additional funding across numerous use cases.
I divide them into three areas now. We have our direct to client work, so that’s an AI that’s exposed directly to end users. There’s coaching use cases, where we’re trying to support frontline workers, community health workers, ag workers, with a coach, supervisor assistant, not to replace the human.
But to augment that, and we touched a bit on that in episode two, and then also a new use case that we weren’t talking much about, that’s really been quite popular is a kind of program manager assistant. And we break that assistant into kind of three buckets. One is a knowledge assistant, which is what a lot of people picture with a Q and A type bot.
that’s trained on your data that can answer questions, a data assistant that can interpret and analyze and help understand data that you might be dealing with as a program manager, like which county has the highest burden of disease. And then a workflow assistant. If you’re reviewing documents or moving documents across teams, how can you do that?
Imagine a program team of 10 people who’s running a epidemiological program or surveillance program. An AI that can really help all 10 of those team members is something we’re getting increasingly excited about. So there’s a ton going on both within Dimagi and obviously way more outside of Dimagi, but a lot of progress.
At the same time, I think everybody’s holding their breath on, when’s the next jump in foundational models. So we’re recording this on May 22nd OpenAI just released CHET GPT 4. 0. And Some people were guessing that might be 5, it’s not, it’s a amazing step in some directions, but not a huge step in terms of the next level of foundational model.
And one of the interesting things that’s hard to put it in context, the jump that Anthropic just made so they, a month or two back released Cloud 3, which was, a massive difference between Cloud 2 and Cloud 3. And people now view it as on par with. OpenAI, ChatGPT4. So one, people are excited there’s some competition because OpenAI’s ChatGPT4 is pretty far out ahead.
But two, the fact that other companies are replicating these massive step changes. And Performance and Capabilities is also I think giving to me some credibility to the people who think we’re in a very fast curve right now and just have to be patient. There was a podcast that we can link to in the show notes from the co founder of Anthropic with Ezra Klein from the New York Times.
And one of the interesting things on that podcast I found was, he’s look, if you’ve been on the inside of this, we’ve been seeing, really impressive step changes for years. And so the fact that the next one hasn’t come out in months doesn’t bother us, but to consumers who like just got exposed, saw this huge jump between 3.
5 and 4. Now it feels weird that you haven’t seen that next jump, but they’re all like no, this is like nobody inside this world is worried about the progress. And maybe that’s, salesmanship or wishful thinking and people are worried, but his view is like to the outside people who have not been doing this for a decade.
It feels maybe slow, given how fast 3. 5 to 4 was, but it’s if you’re inside and you’re testing these and you’re like one of the engineers, you’re like, you’re just, this is. This is just how R& D works. We’re moving forward and some huge things can come out next.
Amie Vaccaro: Jon, I don’t think I’ve heard anyone say this feels slow, but that’s funny, a funny perspective. Stepping back a little bit, Jon, I’m curious to hear, and maybe this is for the audience too what do you see as Dimagi’s role in AI? And clearly like we’re not building foundational models, right?
We’re not trying to compete at that level. So as a tech company in global health, like what do you see as our most important role here?
Jonathan Jackson: Yeah. I think we, and Brian in particular and the team that he is leading, we think a lot of this is very aligned with our core philosophy and ethos as Dimagi, which is there’s these amazing technologies out there, and technology is going to keep getting better and markets are going to keep driving it into.
Profitable use cases, and we see huge potential and with AI potentially transformative potential to make it also work for The impact use cases that we care about. So what we’re trying to do is understand where the market’s going to take care of itself. And obviously we’re not gonna influence that.
This is well beyond the resources the development sector mobilizes or is even influential in, but there’s a lot of people who are concerned about equity within use of AI. There’s a lot of people concerned about how this will be a race to a monopoly, either at the government level or at the corporate level, and how will AI be.
Equitably accessed and equitably deployed. And so that’s really what we’re focused as saying, how can we as quickly as possible, as safely as possible, and obviously ethically test these use cases, show our partners what’s possible, show governments what’s possible, just get this tech into the hands of people.
And And for us, that’s been one part becoming expert and just what the tools and technologies can do, making sure we’re doing it, not just with any one proprietary model, but across all the models, understanding where open source models are. And for us, our OpenChat Studio platform is about just making it easy to learn and test.
So we have, hundreds of bots we’ve built at this point, hundreds of use cases. We’re going to probably build thousands and not the two near future. Many of them are going to be totally useless. Not work at all, either because the AI didn’t work or the use case wasn’t worth trying to solve.
But a couple of these are going to work and they’re going to work really well. And they’re hopefully going to show a path on what use cases are possible today, what people should be thinking about for tomorrow. And I think that’s a really important role that we play. And I think we’re uniquely suited to play it given our.
20 years of experience, our global presence the fact that Brian has a research team with research backgrounds, the fact that we have program people who understand the day to day of what it’s like to be CHW and how to support program managers, former government officials, partnering with government.
So I think you need to really understand a lot to know where you can add value from an equity lens in these use cases. And I think we’re very fortunate that across our team we do have that collective skill set.
Brian DeRenzi: I, I think to every point, I have something to add and I’ve already forgotten half of them, but if I were to jump in, I would just echo the team perspective that we have an incredible team, a lot of the projects that the research team are focused on are around evidence generation.
So everybody knows that we can. generate interesting text using large language models, but there’s an open question whether any of these use cases that we’ve come up with can lead to a meaningful impact. Can we move the metrics and the indicators that we care about? And so we, we have a handful of different projects where we’re trying to do that across a range of different scenarios where we’re actually going out where we’re deploying these things in a meaningful and rigorous way and trying to evaluate whether we can.
We can shift important indicators and in global health and mental health across the board. And our team is not only engaged at that level, but and has all the experience that Jon mentioned, but we also internally have an incredible range of languages that we speak as a company.
And we did a little exercise. While you were away, Amie, where in a literally like a four hour period or something, we tried out over a dozen different languages on a model and got some early early understanding of how well the model could communicate, could understand, it could generate text across those different languages.
And it was really, it was like a fun few hours where, we had people jumping in saying, Oh, I speak I speak Zulu and I speak Chichewa and I speak Wolof and I speak whatever and so we were like spitting up all these different bots and they were engaging with them and giving some feedback and like filling out these forms and, when the dust settled, we had this big spreadsheet that, that kind of gave us some initial understanding of how well a few different models were working across these languages.
And it was really a a fun moment where we got to leverage the incredible diversity and the rich backgrounds of all the individuals that make up Dimagi. And so I think we’re really well positioned as a team to be able to take this on and push this work forward.
Amie Vaccaro: I really appreciate just hearing a bit about the work that you guys are doing around language, because at the most fundamental level if these models don’t speak your language, you’re not going to get any benefits from AI, right? That feels like such an important layer that we can bring in and one of one of many.
I want to maybe provide a little bit of a framework for the audience in terms of how, like, how are we thinking about bending the arc of AI towards equity? And Jon, you had mentioned three different areas where we’re seeing funding, right? Direct to client work, coaching, and then kind of program manager use cases.
But what I want to. Frame is maybe even higher level, like the three kind of areas where I see the team’s applying effort. So one is that direct to clients, right? How can we, how can AI directly work with end users and support them the ways that, that we’re using chat GPT daily? I don’t know about you guys, but I am.
The second being, how can AI support healthcare workers? So that’s where that coaching use case you mentioned and even, potentially the program manager use cases could fall in, but knowing how essential frontline health workers are to our end goal, right? Dimagi’s end vision is a world where everyone has access to services that they need to thrive and health workers are critical there.
It can’t just be AI bots. So how can AI really enable and equip frontline health workers in all their various forms? I think that seems like the second sort of bucket of where we’re investing. And then the third bucket. is really looking at the ecosystem of AI and how it’s being developed.
And that’s where Jon mentioned OpenChat Studio, which I’m not sure if we’ve talked about on this podcast yet, but it’s a developer platform for building bots you can do it with little tech skills, and Brian, I’ll invite you to share a bit more about that in a moment, but I see a lot of work being done at that ecosystem level.
How can we ensure that the testing and building and iterations and these learning cycles that are required are being approached equitably and inclusively so that more folks can be in their building and testing and learning? And it’s not just concentrated in Silicon Valley where that’s happening.
So I’ve been really excited to see some of the progress on OpenChat Studio where you’re bringing in many partners, individuals to empower them to test and learn in this environment. Brian, would you care to speak a little bit to those three buckets?
And then if you want to share a bit more on OpenChat Studio?
Brian DeRenzi: Yeah, it’s a big question and, we could spend an entire episode on probably any one of the three topics there, but I think for the direct to consumer, I think in some ways it’s the most straightforward because there’s an individual, they’re trying to get some information or share some information or we’re trying to make sure that they have access to to the support that they need to accomplish something.
And so we, we have a number of different Projects where we’re doing that. And the projects are starting to diverge in interesting ways, which is good. In some projects we’re focusing much more on language. So if we’re working in a low resource language, making sure that It’s interacting.
And, we have a project in Kenya, for example, where we’re interacting with the youth and it’s important that we’re doing everything in Shang, which is this code mix, language between Swahili and English has lots of slang words thrown in. And even as we push on that, we’re. We’re becoming little mini linguists ourselves within the project, because even the Kenyan teammates we have who are working on this project they’re learning more about Shang than they ever knew before.
And, we’re continually having to refine what it means to speak Shang or to communicate in Shang, if we were to come up with an English equivalent, you can imagine somebody speaking with the Queen’s English and some very proper grammar you can imagine the way that we all speak colloquially and other versions of that and trying to hit the right level of informality and formality of that language
but still make it accessible to people and still make it, approachable enough that.
They’re willing and able to engage with it and engage with the content. So I think those are some of the challenges around language that we’re thinking about in different countries and for different projects from the health worker perspective, the way I’ve been conceptualizing it is, there are end users and then we might have, frontline health workers, community health workers at the next level up, and then they have some supervisors and their facility workers at some point all the way up.
To county and district and national level governments and things. And at every level, you can imagine creating chatbots to support the person exactly at the level and to facilitate the communication between the levels. So for the health worker, You can imagine a coaching tool that’s just for them, nobody else is involved, is a reference, it’s reference material or it’s some skill building material and they can engage with it on their own terms.
You can also imagine if you have a frontline health worker who’s going and doing health visits. Home visits for let’s say pregnant women. Maybe she drops off a digital assistant that’s going to be directed to client and reports back to the health worker.
So now we’ve given the health workers some additional tools to keep track of all of her clients to make sure that she’s able to provide continuity of support between those home visits. She’s able to triage a bit better because she can see who’s having issues or not. And then you can also imagine the version above where it’s looking at the data and looking at the home visits she’s doing, looking at the forms she’s filling out, looking at the services she’s providing.
And. Summarizing that or providing some feedback on how that’s going and identifying proactively some areas to, to support her. And in some versions, we might also communicate that up to the supervisor. So the supervisor can provide better human support to, to that community health worker. So I think there’s a whole range of different things that we can do around a single health worker.
And then for the ecosystem, I think there are a few interesting things. I met one of my colleagues on the research team on the plane and we were on the plane with no wifi and I opened it up and said, Hey, look at this.
And I pulled up Lama three and I had Lama three running on my. Several years old MacBook, and we were making chatbots just for fun offline with an open source model, and it worked surprisingly well in English. When we asked it to speak Swahili, it went into this crazy loop of, it just kept repeating the same words over and over until it, felt like my computer was going to catch fire, so I had to shut it down, we were pushing the limits there, but just the performance that it’s able to do, and this is a quantized version of the smallest model that’s available.
So this is the lowest level of performance you can expect out of the model, and it was able to do. Some of the significant illness guide work that we were doing it was able to do it quite well with no prompting effort at all. So just starting there, I think there’s a lot of excitement around that and, colleagues of ours at Jacaranda Health have already retrained Lama 3 with a Swahili set to get it to speak in Swahili, similar to the work that they did for Lama 2.
So I think there’s a lot of excitement in the like larger ecosystem about open source models and where those are going. Things are getting more powerful. They’re getting smaller. There’s, people who are running entire models just within a web browser, load it into the web browser cache and run it in there.
There’s all sorts of things happening. And so I think there’s a lot of excitement around there and with OpenChat Studio, I think you really hit the nail on the head in that our goal is to support internally our team at Dimagi, but also the larger ecosystem to be able to more easily engage with these models to think about how we can quickly spin something out, spin something up, Test it out on a modality that makes sense for us, whether that’s WhatsApp, or Telegram, or SMS, or Facebook, or Instagram.
So our goal with OpenChat Studio is really to build up the support and to leverage other advances in the open source community to be able to support that entire building and deployment process to, to get something out and into the world and into the hands of people.
Jonathan Jackson: just to build on that last point, Brian, Amie, you mentioned it as a developer tool, which it definitely is. And we have people self hosting OpenChat Studio, but we also took the ethos we had with CommCare, which is, non developers should be able to use this. And not that it’s like trivial, but like with some effort be able to rapidly deploy.
Chatbots or AI use cases. And so we have lots of kind of non technical people on the platform building and testing as well, which, I think is, it’s a very obvious idea to build a platform that lets you build chatbots. Like when we were first starting open chat studio, we’re like, this is the least interesting idea you could come up with for AI, but every little thing adding the safety layer, we added making it easy to connect to Proprietary or open source model out there, making it easy to test multiple instances.
One against Lama, one against OpenAI making it talk to both Telegram and WhatsApp and SMS and have a web version. All these little things add up. And so I’m really excited. Just six to 12 months ago was like, I don’t know, should we build this? And is it an internal thing? And do we need it?
But there’s just so many one off things one has to do to make the user experience for these things good. And to make testing them interesting and easy, any one step isn’t that bad. You could download all the data, put it into a spreadsheet, do a pivot table, and then compare the data. But it’s like each one of these things adds up to be like a big barrier in closing that last 10 percent between making it.
actually work for the users we care about and not. And we saw that with CommCare. We spent tons and tons of time and Brian has an episode speaking about his experience early with CommCare, but getting the multimedia to load correctly and the images to display correctly was like the difference between a low literate user being able to use CommCare and not.
And so these last 10 percent user experiences, Really are like gotchas in, in my opinion, in equitable deployment of technology. And so that’s an area that I’m really excited about. I do think there’s something here with the platform that it just, it’s solving so many annoying edge cases for you that it’s really an accelerant for developers and organizations to use, to be deploying AI.
Brian DeRenzi: I think my hopes and dreams for, or the vision that I have for OpenChat Studio is that it’s a platform that supports the entire process of exploring what we can do with an AI application and rolling it out and deploying it. And there are a bunch of things along the way, including. Needing to build in safety layers or needing to build more sophisticated architectures for different agents as Jon was talking about, or needing to build up some testing infrastructure to increase the confidence or generate synthetic data for that testing infrastructure to increase confidence of the applications that we’re building and the chatbots that we’re building.
, how do you actually deploy that and how do we test that? How do we make sure that it’s working in different languages? How do we capture the nuance and the contextual? Information for any particular use case, one of the early tests that we were doing in our work in Kenya, one of our colleagues was like, Oh I’m running low on money.
I don’t know what to do. I’m not sure how I’m going to eat next week. What should I do? And the chatbot responded, Oh, you should go to a soup kitchen. And she was like, yeah, that’s a great answer if you live in New York, but maybe doesn’t apply to the context where we’re working in Kenya.
I think that’s the vision that I have of being able to really Support that for a large number of people, a large number of different organizations, be able to give access to locally led organizations, be able to give access to, a lot of the work that we’re doing we’re trying to get the partners involved because there is some democratization, let’s rephrase that, there is some What’s that?
How do you say that word?
Jonathan Jackson: democratization, this is staying in, by the way.
Brian DeRenzi: Good. I’m glad my linguistic anyway, fits the theme. So I think there is some like democratization of large language models where all of the prompts are just written in plain text. Opposed to needing to learn programming languages , but just like general, Programming and approaches to, to building things.
You’d have to learn some programming language and kind of upscale around all that before you can do it. We’re trying to be very intentional in the projects that we’re doing and bringing partners into the fold and getting them involved in writing prompts and seeing how we’re writing prompts and seeing how it affects things in order to spread that that ability to bend and manipulate large language models to, to their contexts and to their environments.
Amie Vaccaro: It’s like large language models in some ways are democratizing AI because it’s more accessible. And then Open Chat Studio is taking that a layer further, right? Democratizing the ability to be building these chatbots in a safe environment with a lot of thought put into how can we best be building, testing, learning, and even deploying these chatbots into real world use cases.
One thing that is coming to mind for me is I heard a quote somewhere where AI is changing everything and it’s foolish to go back to day to day operations because things are changing so fast.
Amie Vaccaro: And so I’m curious. Jon, what are your thoughts around how should we be thinking about AI with our existing offerings, right? CommCare, Assured, HERE how are we thinking about including AI in those?
Jonathan Jackson: Yeah, it’s a great question. I was just talking with a potential partner this morning, right before this podcast, and I’ve given this advice to many organizations who are not going to develop their own AI products, but are thinking about how AI can be used in their work. And there’s like the enterprise.
Software that we use as an organization, whether that’s Salesforce or Google, Gmail or Tableau and Power BI and things. And those are going to have their own kind of company wide capabilities that we’ll just start using and companies can choose to adopt them quickly or slowly just like they could with internet technologies and IT technologies.
But like eventually this is just going to be table stakes for how organizations operate, right? There’ll be AI infused capabilities in all these products. Then you see add on models where they’re like, no, no, this isn’t part of the product. We want to charge you more to get access to this AI. And I’m curious how that market’s going to evolve.
So there’s like open AI, which is 20 bucks a month. To have all the access to a chatbot. But then there’s Google search just has Gemini embedded in it, you don’t have to pay more for it. So there’s going to be, I think, an interesting evolution of what the pricing model is and what the adoption curve is.
And the reason I brought that up is as we think about it for CommCare Insured here, there’s like a muscle model where like, all right, let’s build this new set of features. And try to frame it as an add on that we can charge for. And so the mental model is similar to product development, right?
Can we invest enough R& D to create enough value that we can then recoup it by charging for it? And then there’s a flip side of like generative AI like capabilities are just going to be table stakes and an expectation that they’re in all products and you can’t charge more for them because everybody will have them.
And that could end up being the world we enter as well. Now, Really good generative AI features are not necessarily cheap. So if we end up in a world where this is just expected, shared here has virtual health coaching available and remote patient monitoring. So there’s a video. You can easily imagine adding AI capabilities to interpret the video.
In fact, we’re doing research and development on that now. It’s expensive. You’re talking about tens of cents per analysis. And if you’re doing that on all of your transactions, like these things add up. So we’re looking at three different areas, table stakes features. Like we need to do this just to either keep our competitive advantage or.
We’re worried we might need to catch up to what other tools can offer. Features that are somewhere in between an add on or just part of the product. The most obvious is an AI coach for CHWs. We are exploring whether an AI could look at the app you’ve built in CommCare or look at the workflow you’re doing in Shared here and then create an AI for you that could help coach the workforce that you’re supporting or the end client.
And then the third is like clearly net new things that the products don’t do today that obviously we’d have to charge for if we offered. And we have teams thinking and developing testing features that could be on any of those three dimensions. I will say, because the market is really unclear to me, it’s hard to know how to invest.
As the CEO of Dimagi in those types of efforts. So we obviously are investing a ton in OpenShot Studio and for all the reasons that Brian mentioned. And then within our product lines, we’re doing a lot of testing and iterating, but it’s interesting like, should we build the next obvious feature customers have been asking about for 12 months that has nothing to do with AI?
Or take a leap onto, an AI based feature that were far less clear on the demand and monetization. So obviously to both end, almost every software company is in a both hand state right now. But I think there’s a lot of potential for AI, and I’m interested. A lot. And Brian and I talked about this when we were together in, in Oxford a couple weeks back, I’m really interested in voice interfaces as well, which is doesn’t fit into any of those three buckets we talked about, but like for CommCare, you enter data into a mobile app, it takes you through a decision support algorithm, you’re collecting data during the encounter.
You could easily imagine replacing that with just. Voice conversation between Brian and I, and then the AI just interprets all the data out of that conversation. Or you can imagine after I talked to Brian, I just quickly dictate for 60 seconds and Brian actually sent me a quick demo of this right after we talked demonstrating, just like giving a summary.
With errors and like in totally normal human Hey, this child has 17 beats per minute, et cetera. And like the AI is very good at extracting the data model out of that type of conversation. And so I don’t think we could charge for that. Like, you know, That’s just kind of so it. Tangential to how we think about our markets and our business models for these products right now, that some of the really interesting step changes and the user experiences you can create, the approaches you can create, they’re really hard to think about strategically, for us as a company, because it’s just really unclear, like what is the payback of that, even though the benefit to the end user is really obvious.
So we’re definitely going to be exploring a lot with voice interfaces, but I don’t. I don’t think we can charge more for the voice interface in CommCare, if it works, it’s gonna be like part and parcel of hopefully how CommCare works, one or two years from now. So lots of really exciting stuff.
And then there’s the stuff that people wanted to have done this whole time, even pre COVID. Generative AI exploding. Like it, it has always been a goal that you can do predictive analytics with Comcare data. The shared tier team has always had that kind of video interpretation question of can you automatically detect whether somebody is ingesting a pill in the video?
So there’s kinda like the. Use cases that have been around for a long time that are now maybe a lot easier to test or a lot easier to deploy and that’s really exciting too. So, um, there’s a, There’s a lot we’re thinking about and it’s interesting cause like, it’s really hard to know what is going to end up having, there’s tons of value.
And then Brian and I were talking about this too. There’s we’re, Dimagi is extremely good at creating impact and creating value. What’s very hard is knowing what will the market accept that it’s willing to pay for? About that impact and that value. And as we’ve talked about at length on this podcast and in a lot of our public documents, like there’s unfortunately a very low correlation between what the market and the development sector is willing to pay for and the impact it generates sometimes.
So a lot of it’s going to come down to framing, right? What, what feels okay for people to be paying for and how much of that is where we think the most impact and the most equity can be generated.
Amie Vaccaro: I’m curious to hear from both of you, your overall feeling about AI right now in terms of I feel like there’s So much potential and it’s a bit of a nervous system overload situation where there’s just so many possibilities for how we can be thinking about it and applying it.
And I’m thinking about AI for, my day to day work, AI for our products, AI for new products, like so many layers and process of choosing is really tricky. So I’m, I think I’m in a place of cautiously optimistic, but also maybe some overwhelm. What about you guys?
Brian DeRenzi: Yeah, maybe I can go first. I think that makes sense. There’s a lot happening. A lot of different players and Jon raised the point earlier, in, in some ways 12 months ago, it was easier. There was one model that was clearly better than all the rest, GPT 4. And if you wanted, to do the most sophisticated thing, or you wanted to work in the largest number of languages, you had one option, which was to work in and build off of GPT 4.
And I think it’s exciting. There’s some excitement that we now have a number of large foundational models from multi billion dollar companies. LLAMA 3 is much better than LLAMA 2 and apparently the 400 billion, the most impressive version is still training.
So that’ll get better. GPD 4. 0 is a much faster, much less expensive version that, That is on par and, it seems to smooth out some of the rough edges of GPT 4 in our early testing.
I am optimistic and I’m excited about the work that we’re doing because I think we’re keeping one eye on that and trying not to get sucked into the vortex of, what AI, what major AI update happened this week versus last week and letting that derail us. But we’re really focused in the team on exploring a range of different use cases and around generating evidence and convincing ourselves that we can actually move important indicators.
Can we increase the self efficacy of a young person in Kenya to make choices about her own sexual and reproductive health? That’s what Dimagi is here to, that’s what we’re here to do is to support things like that. I’m excited about the focus that we have on that kind of work and around trying to generate that evidence and take that effort forward.
I’m unsure where the future. is going. All of the, multi billion dollar companies seem to be focused on multimodal different people at different times have talked about Oh, maybe the future is in smaller, more targeted like the, Very focused medical models are very focused lawyer models or something like that.
And I wonder whether some of the sort of unintentional benefit for lower income markets goes away with that. But I’m also optimistic because we’re starting to see better performance out of Smaller models and better performance out of open source models. And we’re exploring a wide range of use cases, but like narrowing in on trying to evaluate and generate evidence around whether we can move.
The metrics and indicators that, that really matter in, in our field.
Jonathan Jackson: Yeah, it was really well said Brian. And I think for me, it’s definitely. Optimism is the only viable position to take, given that it’s going to keep moving fast. So we need to think about what are the positive benefits of it. I think there’s huge negative externalities we’re going to well, I mean, everybody does.
This is like, there’s gonna be plenty of downsides. But as Brian said, there’s lots of reasons to be excited that there’s going to be. Unintended positive externalities that are just going to come out for free because that’s just like the natural step of evolution of some of these models and approaches.
So I’m really excited by that. And I think we talked about this on episode one of our AI series, but I still worry as these models get better and as you can be 70 percent good enough. Is that going to cripple innovation on being like really good at anything? So I had a interesting conversation, one of our new product lines from like, you are not allowed to hire a human support person as an, as a thought exercise, right?
Just accept whatever the AI can do today, assume we’ll get better tomorrow, but like never hire a human for the support team on this new product. And then we were talking to him I, this might be the right, forcing function for us to try it because it’s low stakes. The support burden is very low on this new product.
And it’s interesting to think through things like that. And you’re like if we just accept that’s what we can get today and we hope it gets better tomorrow, that might be a really good business decision and maybe even like an optimal human, use of time decision. But what if it never gets better than 70%?
And then you’re like, Oh, and like for support, maybe that doesn’t matter, but for coaching health workers or for being empathetic to people seeking sexual, and reproductive health issues, we shouldn’t settle for 70%. And we may enter a world, particularly with the next evolution of a lot of these models, where it’s like clearly able to get to 70%.
And then you’re like, Oh crap, what are we trading off by just jumping onto this? And obviously like we are going to jump onto it. We’re not going to stop ourselves. So that’s one of the things I think about that’s both a optimistic view that like we’re getting there. We’re getting to 70 percent pretty rapidly, but then also what are the implications of that and how confident we are?
We’ll get, maybe we’re going to get to 150 percent by jumping on at 70 and it keeps getting better, but maybe it stops and. AI in general won’t stop, but stops for the use cases that we care about. So that’s one thing that I do think about, but I think there’s so much uncertainty ahead and so much potential for the benefit of these tools, particularly in areas where the alternative use is not.
Like we we Dimagi cannot afford to invest enough to provide perfect support for any of our products. Like it’s just too expensive. And it’s really exciting. When you think about different use cases like this and the potential for it, even if they’re not perfect today, if they’re on the curve and getting better tomorrow, that’s just such a powerful place to be.
And so I leave with optimism, but it is. And increasingly challenging. One of the really exciting things Brian’s working on is like, how do you just compare the performance of these things today? And then it’s obviously going to change tomorrow, even within the tools.
, I just want to frame the 70 percent question, I think in the vocabulary of our first episode was avoiding the dystopia where, only the rich people get to see the real live, health provider or mental health specialist or whatever. And everybody else is left with that 70 percent is good AI version.
Brian DeRenzi: And so I, I think it’s really important that any of the optimism or any of the excitement or any of the work that we’re doing we’re holding that in our mind at the same time and actively working to avoid it. We’re actively working to increase the equity and exposure and access to these tools.
And we’re also keeping in mind That dystopia that we want to avoid so that we don’t unintentionally build solutions that, that kind of push towards that. It’s it’s a a difficult problem in that there’s no clear answer for how to proceed. But yeah, I think incredibly important to keep in mind.
Amie Vaccaro: absolutely. Yeah. And Brian, thank you so much for tying it back to that original question of, yeah, how can we avoid this dystopian future where only high income people in high income markets have access to real health workers, right? And there’s so much to be mindful and thoughtful of and just.
Really grateful to hear from both of you on all of the work that’s been happening and all the thought that’s been going into this. We’ll definitely have to circle back in a couple months. See where things are at. Things are moving so fast. Thank you both so much.
Brian DeRenzi: Thanks, Amie.
Jonathan Jackson: Thanks Brian. Thanks Amie.
Thank you so much to Brian for joining us today. My biggest takeaway is that AI is moving fast. It needs to be thoughtfully stewarded to make sure it’s directed towards the most impactful use cases. We need to do so being fully aware of the risks and pitfalls. Since recording this episode, I had two ahas. I wanted to share.
The first a hot is an analogy that the open chat studio team shared with me. . An LLM is a kin to a brain that has no memory. All the LLM brain can do is process whatever input it gets through the nervous system and generate responses based on that input. Open chat studio is like the body that compliments the LLM and creates a useful organism.
Open test to do provides everything else. Domain specific knowledge and experience guardrails, supporting systems, et cetera.
The other aha I had was around how we can think about technology like AI within our core products as Dimagi.
And this came from a conversation with a director of product on our team.
Products exist to solve a problem. The evolution of AI doesn’t change the problem that our customers and our clients are facing. If AI is the best way to solve a problem, then great. Let’s use it. But let’s not use technology just for technology’s sake. Let’s stay focused on the problem and the best ways to solve those problems and be open to exploring the various ways technology can be applied to those problems.
That’s our show.
Thank you so much for joining. This show is executive produced by myself, Michael Keller. Her is our producer and cover art is by Sudan, Chicano.
Other Episodes
Meet The Hosts
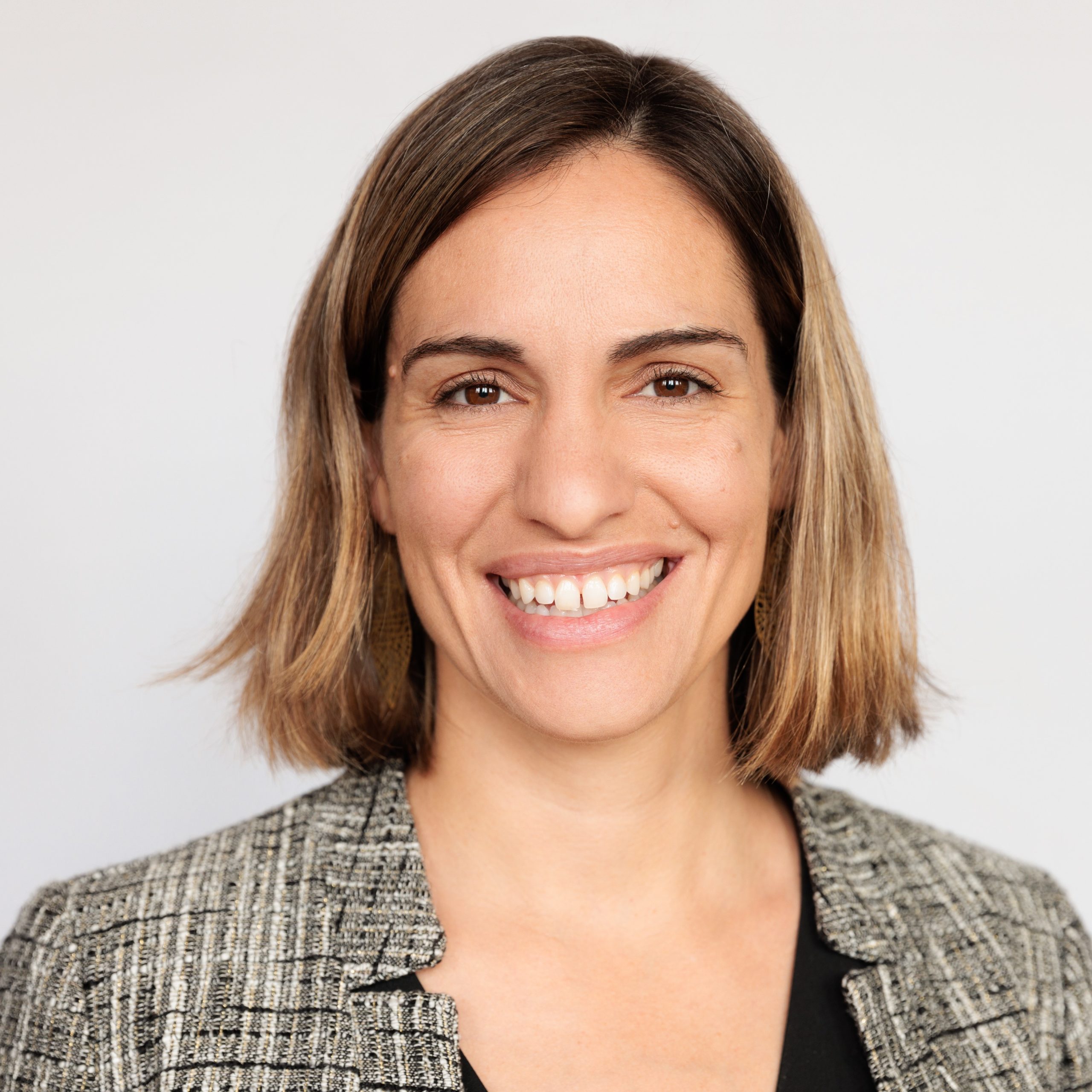
Amie Vaccaro
Senior Director, Global Marketing, Dimagi
Amie leads the team responsible for defining Dimagi’s brand strategy and driving awareness and demand for its offerings. She is passionate about bringing together creativity, empathy and technology to help people thrive. Amie joins Dimagi with over 15 years of experience including 10 years in B2B technology product marketing bringing innovative, impactful products to market.
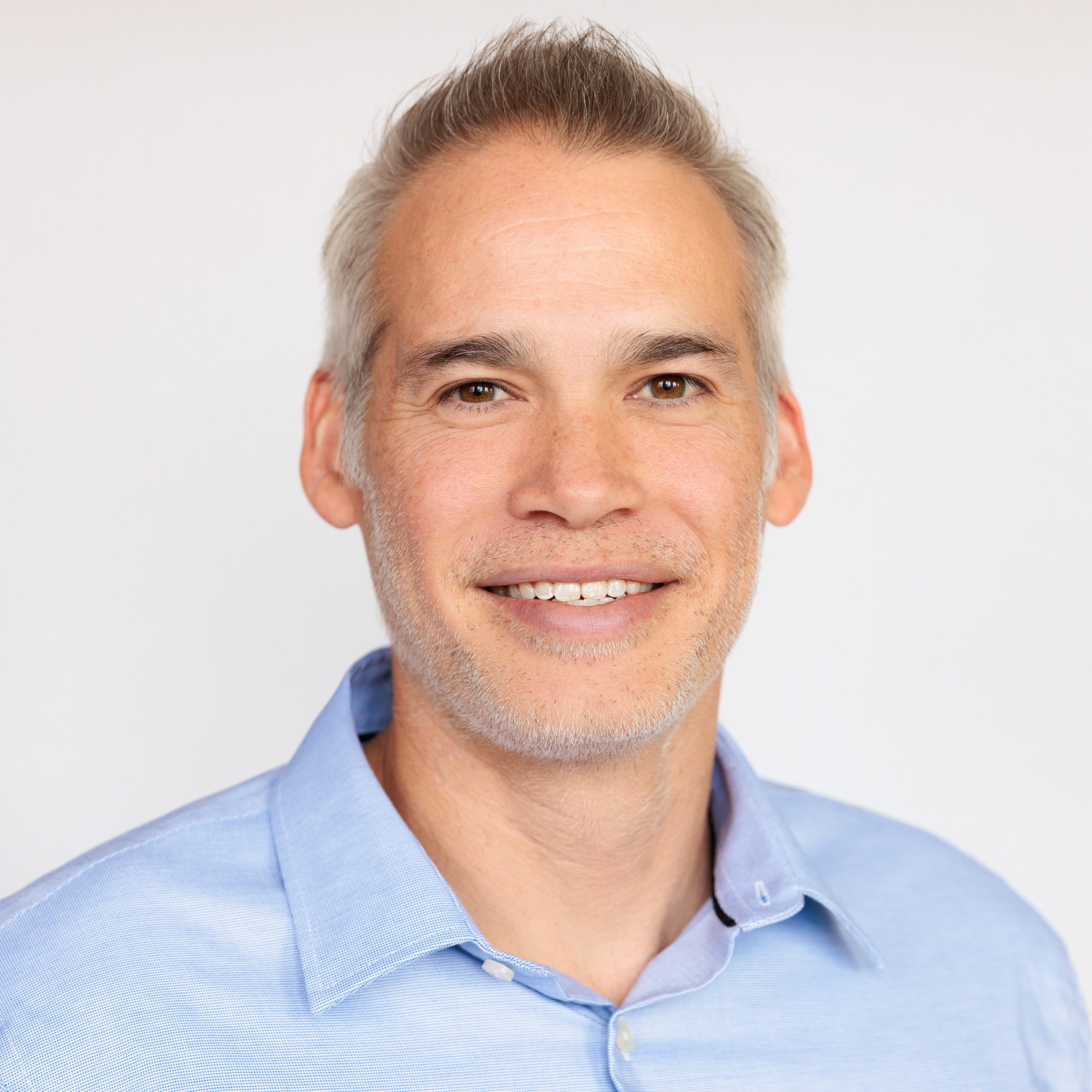
Jonathan Jackson
Co-Founder & CEO, Dimagi
Jonathan Jackson is the Co-Founder and Chief Executive Officer of Dimagi. As the CEO of Dimagi, Jonathan oversees a team of global employees who are supporting digital solutions in the vast majority of countries with globally-recognized partners. He has led Dimagi to become a leading, scaling social enterprise and creator of the world’s most widely used and powerful data collection platform, CommCare.
Explore
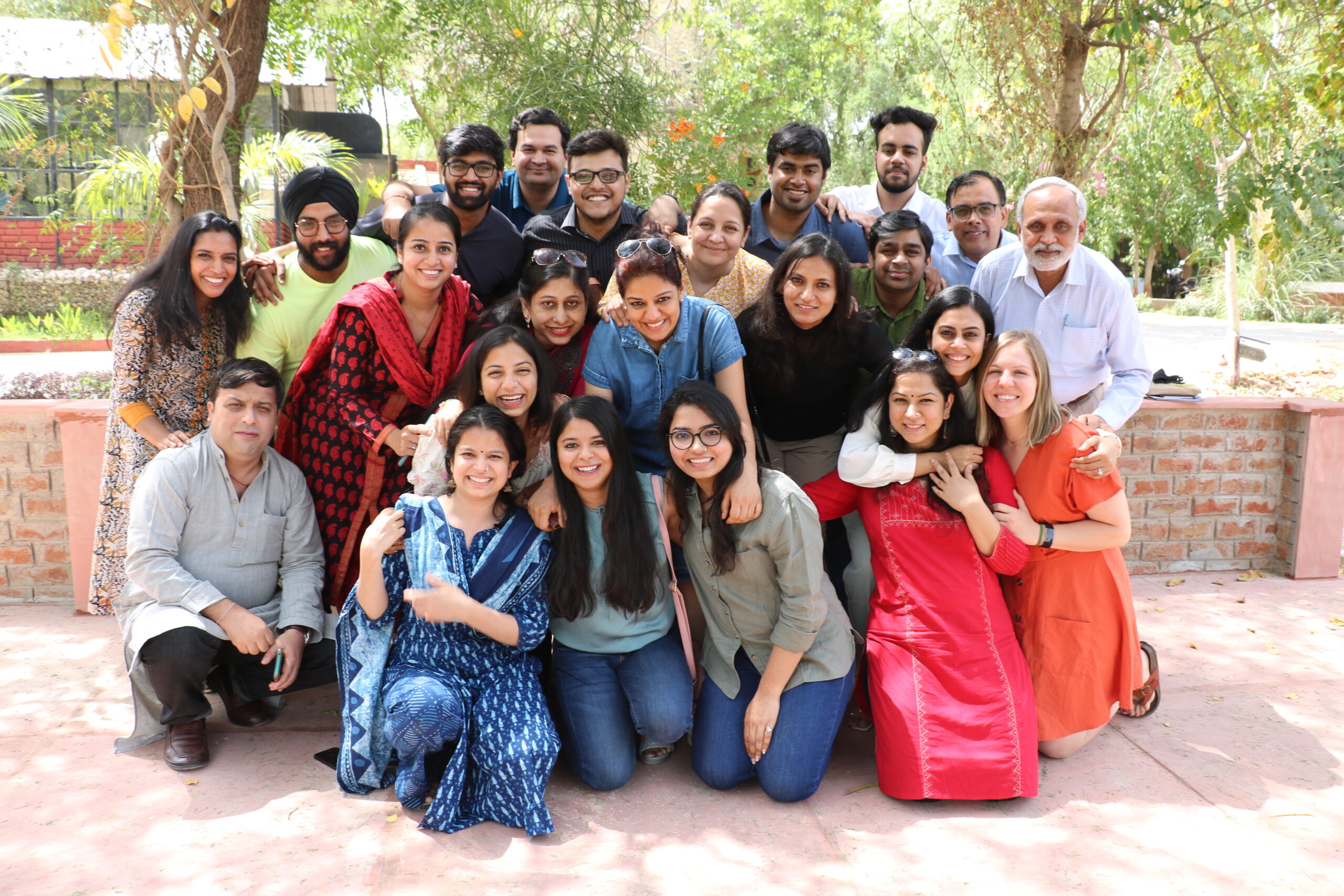
About Us
Learn how Dimagi got its start, and the incredible team building digital solutions that help deliver critical services to underserved communities.
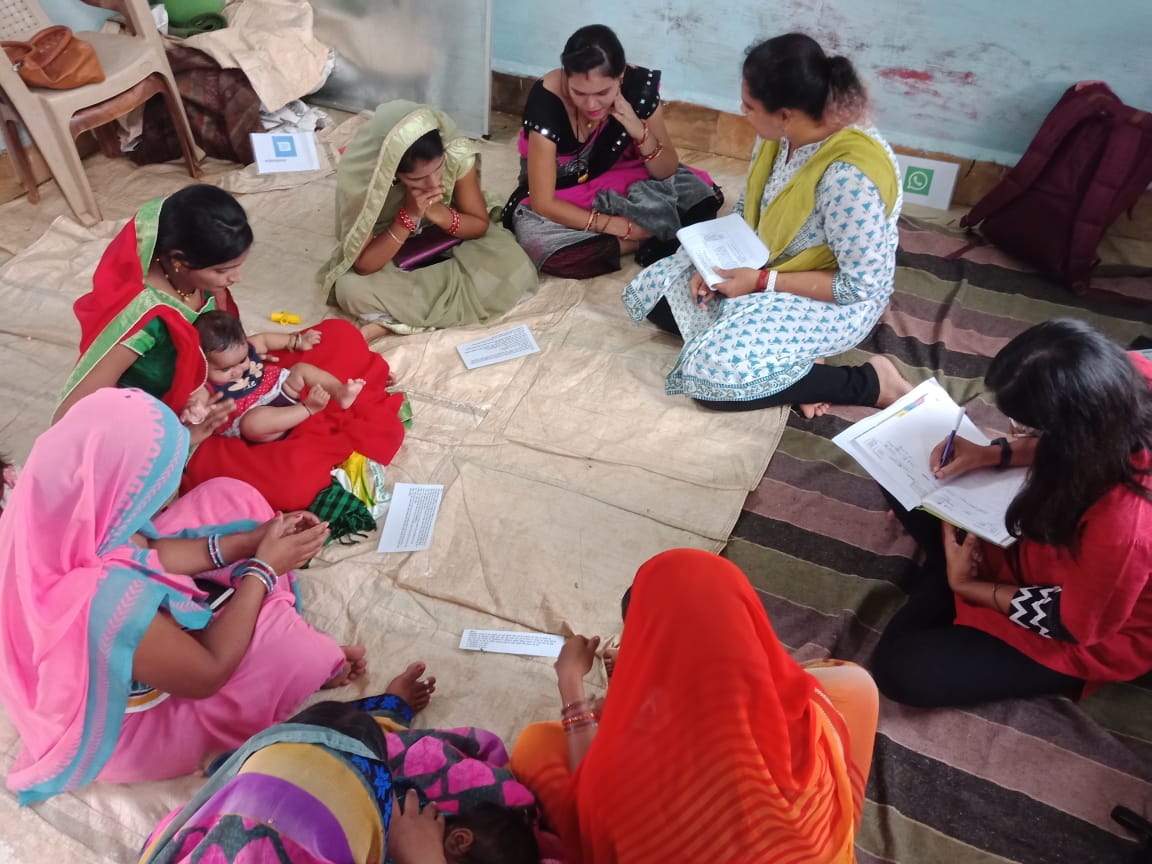
Impact Delivery
Unlock the full potential of digital with Impact Delivery. Amplify your impact today while building a foundation for tomorrow's success.
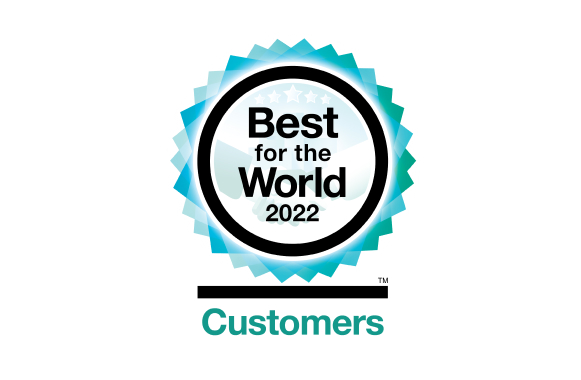
CommCare
Build secure, customizable apps, enabling your frontline teams to collect actionable data and amplify your organization’s impact.